Modeling Health Outcomes with Spatial Multilevel Methods
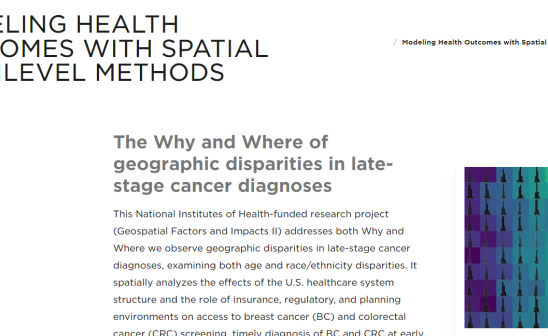
The Why and Where of geographic disparities in late-stage cancer diagnoses
This National Institutes of Health-funded research project (Geospatial Factors and Impacts II) addresses both Why and Where we observe geographic disparities in late-stage cancer diagnoses, examining both age and race/ethnicity disparities. It spatially analyzes the effects of the U.S. healthcare system structure and the role of insurance, regulatory, and planning environments on access to breast cancer (BC) and colorectal cancer (CRC) screening, timely diagnosis of BC and CRC at early stages, and patterns of geographic disparity in BC and CRC prevention and outcomes (i.e., cancer stage at diagnosis) by race or ethnicity, sex, and age. Since this analysis requires spatial advances in multilevel modeling we are developing new spatial analytic features to handle spatial relationships in multilevel data. This functionality will be incorporated in our free and open-source software, which will allow any researcher to conduct sophisticated analyses across multiple scales and spatial regimes.
The impact of state-level insurance and regulations
State-level insurance and health professionals regulations vary considerably across the US, offering the opportunity to study their impacts on health outcomes. Previous research in economics has focused on financial or economic impacts. We consider various regulations that could impact the level of information available to consumers, propensity for the health system to improve such information, and constraints on or incentives to providers that could impact preventive care practices and services. We find several regulations are significant predictors of cancer screening and of late-stage cancer diagnosis, which logically follows downstream from screening utilization. We are not able to link health insurance status or type to the cancer registries, because our focus is on the entire population (not a survey sample from it).
Advancing spatial multi-level modeling …
By allowing researchers to explicitly incorporate spatial effects in disparities research within a spatial multilevel modeling framework, spillover effects across counties can be modeled while simultaneously accounting for spatial heterogeneity in the dynamics related to cancer outcomes. Ignoring spatial effects in statistical analysis can not only result in misleading inference but also in omitted variable problems. Our methodological advances contribute to more robust parameter estimates in health evaluation and disparities analysis to reflect the significant variation in predicted outcomes between locations. Instead of relying on single global estimates, researchers will be able to use the spatial multilevel estimators to characterize the relative influence of the correlates of cancer outcomes along multilevel, temporal, and urban-rural dimensions.
… with “big” health data in Research Data Centers
Using available population census data for health is an aspect of ‘Big Data’ that should not be ignored, as capabilities are available in Census, National Center for Health Statistics (NCHS), and Centers for Medicare & Medicaid Services Research Data Centers (RDC) to conduct population research using huge data files. Our work using the NCHS RDC is the first to date that uses the comprehensive database of cancer registries across the US. A major benefit to spatial science research is the availability of spatial analytic and mapping capabilities inside the RDCs, where the best population-based, spatially-sampled data (the US Census restricted-use microdata) are located.
This project is funded by the National Institutes of Health under Award Number 2-R01CA126858. The funding period is from September 2012 through June 2017. It is directed by Co-PIs Lee Mobley, Associate Professor in Spatial Science and Health Economics at at Georgia State University's School of Public Health and Andrew Young School of Policy Studies and Luc Anselin, Stein-Freiler Distinguished Service Professor of Sociology and the College and Director of the Center for Spatial Data Science. Levi Wolf, the Research Assistant on the project, is a Ph.D candidate at Arizona State University and Visiting Scholar at the Center for Spatial Data Science.
For more information and code, see Levi Wolf's:
- post here
- spatial multilevel code
- with a tutorial linked in the document notebook
- the code is also available on the Python Packaging Index and will soon be available under the PySAL umbrella at https://github.com/pysal/spvcm
(Originally Published on 05-15-2017)